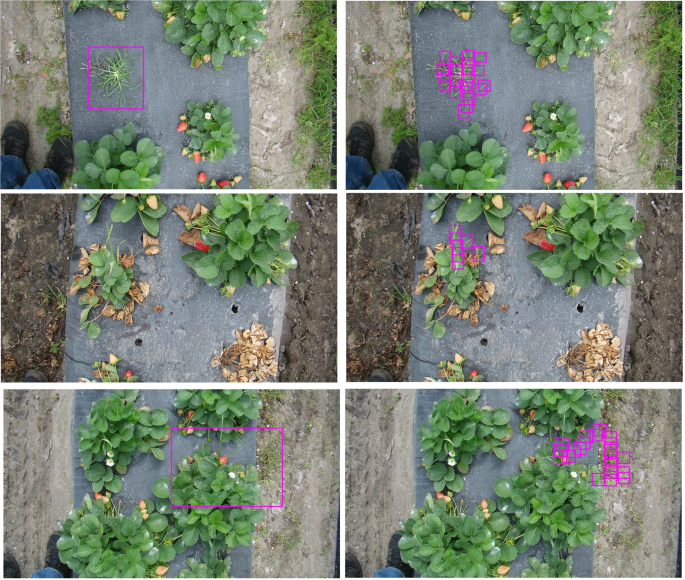
Secretariat, G. Eleusine indica (L.) Gaertn. GBIF Backbone Taxonomy. Checklist dataset, https://doi.org/10.15468/39omei, Accessed 24 April 2019 (2017).
Masin, R., Zuin, M. C., Archer, D. W., Forcella, F. & Zanin, G. WeedTurf: a predictive model to aid control of annual summer weeds in turf. Weed Sci. 53, 193–201, https://doi.org/10.1614/WS-04-066R1 (2005).
Nishimoto, R. K. & McCarty, L. B. Fluctuating temperature and light influence seed germination of goosegrass (Eleusine indica). Weed Sci. 45, 426–429, https://doi.org/10.1017/S0043174500093103 (1997).
Chauhan, B. S. & Johnson, D. E. Germination ecology of goosegrass (Eleusine indica): An important grass weed of rainfed rice. Weed Sci. 56, 699–706, https://doi.org/10.1614/WS-08-048.1 (2008).
Webster, T. M. Weed survey – southern states 2014. Vegetable, fruit and nut crop subsection. In Proceedings of the Southern Weed Science Society 67th Annual Meeting, 288. (Southern Weed Science Society, 2014).
Xiao-yan, M., Han-wen, W., Wei-li, J., Ya-jie, M. & Yan, M. Goosegrass (Eleusine indica) density effects on cotton (Gossypium hirsutum). J. Integr. Agr 14, 1778–1785, https://doi.org/10.1016/S2095-3119(15)61058-9 (2015).
Wandscheer, A. C. D., Rizzardi, M. A. & Reichert, M. Competitive ability of corn in coexistence with goosegrass. Planta Daninha 31, 281–289, https://doi.org/10.1590/S0100-83582013000200005 (2013).
Bewick, T. A., Kostewicz, S. R., Stall, W. M., Shilling, D. G. & Smith, K. Interaction of cupric hydroxide, paraquat, and biotype of American black nightshade (Solanum americanum). Weed Sci. 38, 634–638 (1990).
Buker, R. S., Steed, S. T. & Stall, W. M. Confirmation and control of a paraquat-tolerant goosegrass (Eleusine indica) biotype. Weed Technol. 16, 309–313 (2002).
Fernandez, J. V., Odero, D. C., MacDonald, G. E., Ferrell, J. & Gettys, L. A. Confirmation, characterization, and management of glyphosate-resistant ragweed parthenium (Parthenium hysterophorus L.) in the Everglades agricultural area of south Florida. Weed Technol. 29, 233–242, https://doi.org/10.1614/WT-D-14-00113.1 (2015).
Fennimore, S. A., Slaughter, D. C., Siemens, M. C., Leon, R. G. & Saber, M. N. Technology for automation of weed control in specialty crops. Weed Technology 30, 823–837 (2016).
Schmidhuber, J. Deep learning in neural networks: An overview. Neural Netw 61, 85–117 (2015).
Dyrmann, M., Karstoft, H. & Midtiby, H. S. Plant species classification using deep convolutional neural network. Biosyst. Eng. 151, 72–80 (2016).
Ball, J. E., Anderson, D. T. & Chan, C. S. A comprehensive survey of deep learning in remote sensing: theories, tools and challenges for the community. J. Appl. Remote Sens 11, 042609 (2017).
Gu, J. et al. Recent advances in convolutional neural networks. Pattern Recogn 77, 354–377 (2017).
Yu, J., Sharpe, S. M., Schumann, A. W. & Boyd, N. S. Deep learning for image-based weed detection in turfgrass. Eur. J. Agr 104, 78–84, https://doi.org/10.1016/j.eja.2019.01.004 (2019).
Yu, J., Sharpe, S. M., Schumann, A. W. & Boyd, N. S. Detection of broadleaf weeds growing in turfgrass with convolutional neural networks. Pest Manag. Sci. 75, 2211–2218, https://doi.org/10.1002/ps.5349 (2019).
Dyrmann, M., Jørgensen, R. N. & Midtiby, H. S. RoboWeedSupport – Detection of weed locations in leaf occluded cereal crops using a fully convolutional neural network. In J. A. Taylor, D. Cammarano, A. Prashar, & H. A (Eds.), Proceedings of the 11th European Conference on Precision Agriculture. Adv. Anim. Biosci 8, 842–847, https://doi.org/10.1017/S2040470017000206 (2017).
Sharpe, S. M., Schumann, A. W. & Boyd, N. S. Detection of Carolina geranium (Geranium carolinianum) growing in competition with strawberry using convolutional neural networks. Weed Sci. 67, 239–245, https://doi.org/10.1017/wsc.2018.66 (2019).
Sharpe, S. M., Schumann, A. W., Yu, J. & Boyd, N. S. Vegetation detection and discrimination within vegetable plasticulture row middles using a convolutional neural network. Precis. Agric. 21, 264–277, https://doi.org/10.1007/s11119-019-09666-6 (2019).
Chen, Y. et al. Strawberry yield prediction based on a deep neural network using high-resolution aerial orthoimages. Remote Sens 11, 1584, https://doi.org/10.3390/rs11131584 (2019).
Sa, I. et al. DeepFruits: A fruit detection system using deep neural networks. Sensors 16, 1222, https://doi.org/10.3390/s16081222 (2016).
Fuentes, A. F., Yoon, S., Lee, J. & Park, D. S. High-performance deep neural network-based tomato plant diseases and pests diagnosis system with refinement filter bank. Front. Plant Sci. 9, 1162, https://doi.org/10.3389/fpls.2018.01162 (2018).
Bryson, C., & DeFelice, M. Weeds of the South. Athens: University of Georgia Press. 467 p. (2009).
Urban, G. et al. Deep learning localizes and identifies polyps in real time with 96% accuracy in screening colonoscopy. Gastroenterology 155, 1069–1078.e8, https://doi.org/10.1053/j.gastro.2018.06.037 (2018).
Dyrmann, M., Skovsen, S., Laursen, M. S. & Jørgensen, R. N. Using a fully convolutional neural network for detecting locations of weeds in images from cereal fields. In The 14th International Conference on Precision Agriculture, 1–7. (International Society of Precision Agriculture, 2018).
Blaschko, M. B., Vedaldi, A., & Zisserman, A. Simultaneous object detection and ranking with weak supervision. In J. Lafferty, C. Williams, J. Shawe-Taylor, R. Zemel, & A. Culotta (Eds.), Advances in Neural Information Processing Systems 23. Vancouver, BC, Canada, http://papers.nips.cc/paper/4105-simultaneous-object-detection-and-ranking-with-weak-supervision.pdf Accessed 29 April 2019 (2010).
Buda, M., Maki, A. & Mazurowski, M. A. A systematic study of the class imbalance problem in convolutional neural networks. Neural Netw 106, 249–259, https://doi.org/10.1016/j.neunet.2018.07.011 (2018).
Espejo-Garcia, B., Mylonas, N., Athanasakos, L., Fountas, S. & Vasilakoglou, I. Towards weed identification assistance through transfer learning. Comput. Electron. Agric. 171, 105306, https://doi.org/10.1016/j.compag.2020.105306 (2020).
McCool, C., Perez, T. & Upcroft, B. Mixtures of lightweight deep convolutional neural networks: Applied to agricultural robotics. IEEE Robot Autom. Lett 2, 1344–1351, https://doi.org/10.1109/LRA.2017.2667039 (2017).
Vioix, J. B., Douzals, J. P., Truchetet, F., Assémat, L. & Guillemin, J. P. Spatial and spectral methods for weed detection and localization. EURASIP J Applied Signal Proc 200, 679–685, https://doi.org/10.1155/S1110865702204072 (2002).
Milioto, A., Lottes, P. & Stachniss, C. Real-time blob-wise sugar beet vs weeds classification for monitoring fields using convolutional neural networks. In ISPRS Annals of the Photogrammetry, Remote Sensing and Spatial Information Sciences, 41–48. (International Society for Photogrammetry and Remote Sensing, 2017).
Olsen, A. et al. DeepWeeds: A Multiclass Weed Species Image Dataset for Deep Learning. Sci. Rep 9, 1–12, https://doi.org/10.1038/s41598-018-38343-3 (2019).
Zheng, Y. Y. et al. CropDeep: The crop vision dataset for deep-learning-based classification and detection in precision agriculture. Sensors 19, 1058, https://doi.org/10.3390/s19051058 (2019).
Tang, J. et al. Weed identification based on K-means feature learning combined with convolutional neural network. Comput. Electron. Agric. 135, 63–70, https://doi.org/10.1016/j.compag.2017.01.001 (2017).
Redmon, J. & Farhadi, A. YOLOv3: An incremental improvement. arXiv preprint, 1804.02767, https://arxiv.org/abs/1804.02767 (2018).
He, W., Huang, Z., Wei, Z., Li, C. & Guo, B. TF-YOLO: An improved incredmental network for real-time object detection. Appl. Sci 9, 3225, https://doi.org/10.3390/app9163225 (2019).
Redmon, J. & Farhadi, A. YOLO9000: better, faster, stronger. In Proceedings of the IEEE conference on computer vision and pattern recognition, 7263–7271. (Institute of Electrical and Electronics Engineers, 2017).
Redmon, J., Divvala, S., Girshick, R. & Farhadi, A. You only look once: Unified, real-time object detection. In Proceedings of the IEEE conference on computer vision and pattern recognition, 779–788. (Institute of Electrical and Electronics Engineers, 2016).
Redmon, J. Darknet: Open source neural networks in C (2013–2016), http://pjreddie.com/darknet/ (2016).
Lin, T.-Y. et al. Microsoft COCO: Common objects in context. In Lecture Notes in Computer Science (including subseries Lecture Notes in Artificial Intelligence and Lecture Notes in Bioinformatics), 740–755, https://doi.org/10.1007/978-3-319-10602-1_48 (European Conference on Computer Vision, 2014).
Sokolova, M. & Lapalme, G. A systematic analysis of performance measures for classification tasks. Inf. Process. Manag 45, 427–437, https://doi.org/10.1016/j.ipm.2009.03.002 (2009).
Hoiem, D., Chodpathumwan, Y. & Dai, Q. Diagnosing error in object detectors. In A. Fitzgibbon, S. Lazebnik, P. Perona, Y. Sato, & C. Schmid (Eds.), Computer Vision–ECCV 2012 (pp. 340–353). Berlin, Heidelberg: Springer Berlin Heidelberg. https://doi.org/10.1007/978-3-642-33712-3_25 (2012).
Source: Ecology - nature.com