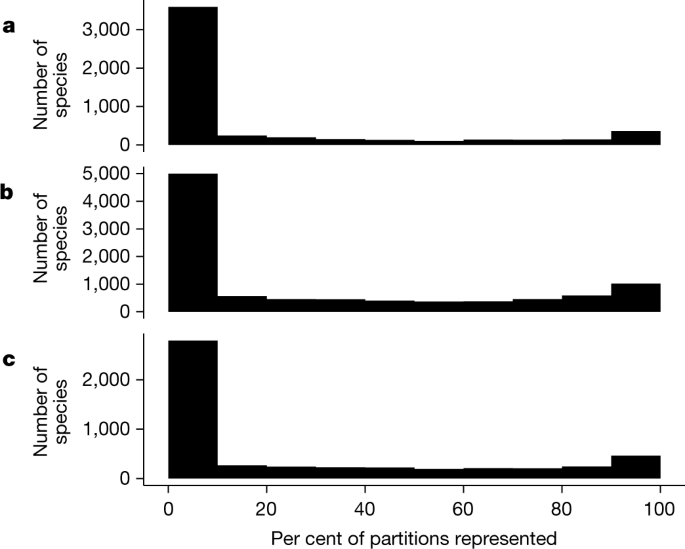
Sgrò, C. M., Lowe, A. J. & Hoffmann, A. A. Building evolutionary resilience for conserving biodiversity under climate change. Evol. Appl. 4, 326–337 (2011).
Moritz, C. Strategies to protect biological diversity and the evolutionary processes that sustain it. Syst. Biol. 51, 238–254 (2002).
Hanson, J. O., Rhodes, J. R., Riginos, C. & Fuller, R. A. Environmental and geographic variables are effective surrogates for genetic variation in conservation planning. Proc. Natl Acad. Sci. USA 114, 12755–12760 (2017).
Laikre, L. Genetic diversity is overlooked in international conservation policy implementation. Conserv. Genet. 11, 349–354 (2010).
Buchanan, G. M., Donald, P. F. & Butchart, S. H. M. Identifying priority areas for conservation: a global assessment for forest-dependent birds. PLoS ONE 6, e29080 (2011).
Beresford, A. E. et al. Poor overlap between the distribution of protected areas and globally threatened birds in Africa. Anim. Conserv. 14, 99–107 (2011).
Rondinini, C. et al. Global habitat suitability models of terrestrial mammals. Phil. Trans. R. Soc. Lond. B 366, 2633–2641 (2011).
Ficetola, G. F., Rondinini, C., Bonardi, A., Baisero, D. & Padoa-Schioppa, E. Habitat availability for amphibians and extinction threat: a global analysis. Divers. Distrib. 21, 302–311 (2015).
Myers, N., Mittermeier, R. A., Mittermeier, C. G., da Fonseca, G. A. & Kent, J. Biodiversity hotspots for conservation priorities. Nature 403, 853–858 (2000).
Scheele, B. C., Foster, C. N., Banks, S. C. & Lindenmayer, D. B. Niche contractions in declining species: mechanisms and consequences. Trends Ecol. Evol. 32, 346–355 (2017).
Butchart, S. H. M. et al. Shortfalls and solutions for meeting national and global conservation area targets. Conserv. Lett. 8, 329–337 (2015).
Vega, G. C., Pertierra, L. R. & Olalla-Tárraga, M. Á. MERRAclim, a high-resolution global dataset of remotely sensed bioclimatic variables for ecological modelling. Sci. Data 4, 170078 (2017).
Venter, O. et al. Targeting global protected area expansion for imperiled biodiversity. PLoS Biol. 12, e1001891 (2014).
IUCN & UNEP-WCMC. The World Database on Protected Areas (WDPA). https://www.protectedplanet.net (2019).
IUCN. A global standard for the identification of Key Biodiversity Areas (version 1.0, first edn) (IUCN, 2016).
BirdLife International. Digital boundaries of Key Biodiversity Areas from the World Database of Key Biodiversity Areas. March 2019 version http://www.keybiodiversityareas.org/site/requestgis (2019).
IUCN. The IUCN Red List of Threatened Species. version 2016.5 http://iucnredlist.org (2016).
Hoban, S. et al. Finding the genomic basis of local adaptation: pitfalls, practical solutions, and future directions. Am. Nat. 188, 379–397 (2016).
Ficetola, G. F. & Bernardi, F. Supplementation or in situ conservation? Evidence of local adaptation in the Italian agile frog Rana latastei and consequences for the management of populations. Anim. Conserv. 8, 33–40 (2005).
Chen, Y. Y. et al. Patterns of adaptive and neutral diversity identify the Xiaoxiangling mountains as a refuge for the giant panda. PLoS ONE 8, e70229 (2013).
Ekblom, R. et al. Spatial pattern of MHC class II variation in the great snipe (Gallinago media). Mol. Ecol. 16, 1439–1451 (2007).
Fjeldså, J. & Irestedt, M. Diversification of the South American avifauna: patterns and implications for conservation in the Andes. Ann. Mo. Bot. Gard. 96, 398–409 (2009).
Verboom, G. A. et al. Origin and diversification of the Greater Cape flora: ancient species repository, hot-bed of recent radiation, or both? Mol. Phylogenet. Evol. 51, 44–53 (2009).
Boumans, L., Vieites, D. R., Glaw, F. & Vences, M. Geographical patterns of deep mitochondrial differentiation in widespread Malagasy reptiles. Mol. Phylogenet. Evol. 45, 822–839 (2007).
Lei, F., Qu, Y., Song, G., Alström, P. & Fjeldså, J. The potential drivers in forming avian biodiversity hotspots in the East Himalaya Mountains of Southwest China. Integr. Zool. 10, 171–181 (2015).
Butchart, S. H. et al. Protecting important sites for biodiversity contributes to meeting global conservation targets. PLoS ONE 7, e32529 (2012).
Maxwell, S. L. et al. Being smart about SMART environmental targets. Science 347, 1075–1076 (2015).
Visconti, P. et al. Protected area targets post-2020. Science 364, 239–241 (2019).
Donald, P. F. et al. The prevalence, characteristics and effectiveness of Aichi Target 11’s “other effective area-based conservation measures” (OECMs) in Key Biodiversity Areas. Conserv. Lett. 12, e12659 (2019).
Gannon, P. et al. Status and prospects for achieving Aichi Biodiversity Target 11: implications of national commitments and priority actions. PARKS 23.2, 9–22 (2017).
Runge, C. A. et al. Protected areas and global conservation of migratory birds. Science 350, 1255–1258 (2015).
Coetzer, K. L., Witkowski, E. T. F. & Erasmus, B. F. N. Reviewing Biosphere Reserves globally: effective conservation action or bureaucratic label? Biol. Rev. Camb. Philos. Soc. 89, 82–104 (2014).
R Core Team. R: A Language and Environment for Statistical Computing. https://www.R-project.org (R Foundation for Statistical Computing, 2017).
Pebesma, E. J. & Bivand, R. S. Classes and methods for spatial data in R. R News 5, 9–13 (2005).
Hijmans, R. J. raster: geographic data analysis and modelling. R package version 2.5-8 https://CRAN.R-project.org/package=raster (2016).
Brooks, T. M. et al. Measuring terrestrial area of habitat (AOH) and its utility for the IUCN Red List. Trends Ecol. Evol. 34, 977–986 (2019).
Phillips, S. J., Dudík, M. & Schapire, R. E. Maxent software for modeling species niches and distributions. version 3.4.0 http://biodiversityinformatics.amnh.org/open_source/maxent (2017).
Kawecki, T. J. & Ebert, D. Conceptual issues in local adaptation. Ecol. Lett. 7, 1225–1241 (2004).
Smith, T. B., Kinnison, M. T., Strauss, S. Y., Fuller, T. L. & Carroll, S. P. Prescriptive evolution to conserve and manage biodiversity. Annu. Rev. Ecol. Evol. Syst. 45, 1–22 (2014).
Vega, G. C., Pertierra, L. R. & Olalla-Tárraga, M. Á. Data from: MERRAclim, a high-resolution global dataset of remotely sensed bioclimatic variables for ecological modelling. https://doi.org/10.5061/dryad.s2v81.2 (2017).
Hanson, J. O., Rhodes, J. R., Possingham, H. P. & Fuller, R. A. raptr: representative and adequate prioritizations in R. Methods Ecol. Evol. 9, 320–330 (2018).
Anderson, M. G. & Ferree, C. E. Conserving the stage: climate change and the geophysical underpinnings of species diversity. PLoS ONE 5, e11554 (2010).
Jones, K. R., Watson, J. E. M., Possingham, H. P. & Klein, C. J. Incorporating climate change into spatial conservation prioritisation: a review. Biol. Conserv. 194, 121–130 (2016).
Miraldo, A. et al. An Anthropocene map of genetic diversity. Science 353, 1532–1535 (2016).
Ester, M., Kriegel, H.-P., Sander, J. & Xu, X. A density-based algorithm for discovering clusters in large spatial databases with noise. In Proc. 2nd International Conference on Knowledge Discovery and Data Mining (eds Simoudis, E. et al.) 226–231 (AAAI, 1996).
Fraley, C. & Raftery, A. E. Model-based clustering, discriminant analysis and density estimation. J. Am. Stat. Assoc. 97, 611–631 (2002).
Rodrigues, A. S. L. et al. Global gap analysis: priority regions for expanding the global protected-area network. Bioscience 54, 1092–1100 (2004).
Beresford, A. et al. Minding the protection gap: estimates of species’ range sizes and holes in the protected area network. Anim. Conserv. 14, 114–116 (2011).
Rodrigues, A. S. et al. Effectiveness of the global protected area network in representing species diversity. Nature 428, 640–643 (2004).
Faurby, S. & Araújo, M. B. Anthropogenic range contractions bias species climate change forecasts. Nat. Clim. Change 8, 252–256 (2018).
Pressey, R. L. & Logan, V. S. Level of geographical subdivision and its effects on assessments of reserve coverage: a review of regional studies. Conserv. Biol. 8, 1037–1046 (1994).
Beyer, H. L., Dujardin, Y., Watts, M. E. & Possingham, H. P. Solving conservation planning problems with integer linear programming. Ecol. Modell. 328, 14–22 (2016).
Gurobi Optimization. Gurobi optimizer reference manual. http://www.gurobi.com (2018).
Hanson, J. et al. prioritizr: systematic conservation prioritization in R. R package version 3.0.3.3 https://github.com/prioritizr/prioritizr (2017).
Source: Ecology - nature.com