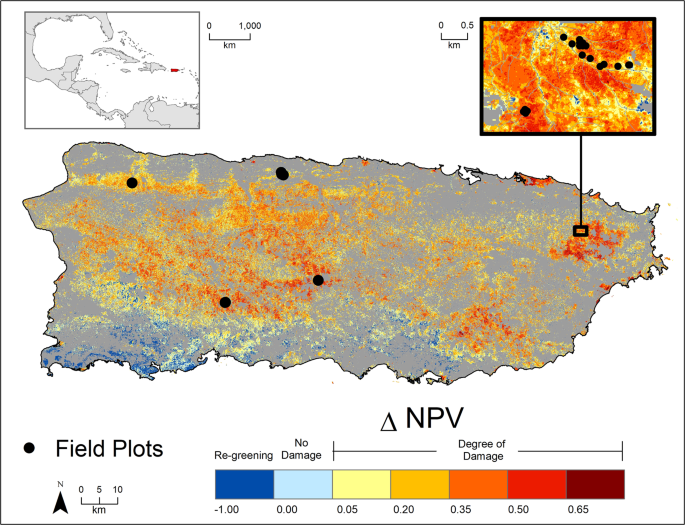
Everham, E. M. & Brokaw, N. V. L. Forest Damage and Recovery from Catastrophic Wind. Bot. Rev. 62, 113–185 (1996).
Mabry, C. M. et al. Typhoon Disturbance and Stand-level Damage Patterns at a Subtropical Forest in Taiwan. Biotropica 30, 238–250 (1998).
Bellingham, P. J. Commentary Cyclone effects on Australian rain forests: An overview. (2008). https://doi.org/10.1111/j.1442-9993.2008.01914.x
Lin, T. C. et al. Typhoon Disturbance and Forest Dynamics: Lessons from a Northwest Pacific Subtropical Forest. Ecosystems 14, 127–143 (2011).
Knutson, T. R. et al. Tropical Cyclones and Climate Change. Nat. Geosci. 3, 157–163 (2010).
Knutson, T. R. et al. Global Projections of Intense Tropical Cyclone Activity for the Late Twenty-First Century from Dynamical Downscaling of CMIP5/RCP4.5 Scenarios. J. Clim. 28, (2015).
Kossin, J. P. A global slowdown of tropical-cyclone translation speed. Nature 558, 104–107 (2018).
Knutson, T. R. et al. Dynamical Downscaling Projections of Twenty-First-Century Atlantic Hurricane Activity: CMIP3 and CMIP5 Model-Based Scenarios. J. Clim. 26, 6591–6617 (2013).
Gutmann, E. D. et al. Changes in Hurricanes from a 13-Yr Convection-Permitting Pseudo–Global Warming Simulation. J. Clim. 31, 3643–3657 (2018).
Fisk, J. P. et al. The impacts of tropical cyclones on the net carbon balance of eastern US forests (1851-2000). Environ. Res. Lett. 8, (2013).
Pan, Y. et al. A Large and Persistent Carbon Sink in the World’s Forests. Science (80-.). 333, 988–993 (2011).
Van Der Werf, G. R. et al. Global fire emissions estimates during 1997-2016. Earth System Science. Data 9, 697–720 (2017).
Chambers, J. Q. et al. Respiration from a tropical forest ecosystem: Partitioning of sources and low carbon use efficiency. Ecol. Appl. 14, 72–88 (2004).
Uriarte, M. & Papaik, M. Hurricane impacts on dynamics, structure and carbon sequestration potential of forest ecosystems in Southern New England, USA. Tellus A Dyn. Meteorol. Oceanogr. 59, 519–528 (2007).
Arriaga, L. Types and causes of tree mortality in a tropical montane cloud forest of Tamaulipas, Mexico. J. Trop. Ecol. Sep 5, 623–636 (2000).
Bellingham, P. J. & Tanner, E. V. J. The influence of topography on tree growth, mortality, and recruitment in a tropical montane forest. Biotropica. Sep 32, 378–384 (2000).
Boose, E. R., Serrano, M. I. & Foster, D. R. Landscape and regional impacts of hurricanes in Puerto Rico. Ecol. Monogr. 74, 335–352 (2004).
Tanner, E. V. J., Rodriguez-Sanchez, F., Healey, J. R., Holdaway, R. J. & Bellingham, P. J. Long-term hurricane damage effects on tropical forest tree growth and mortality. Ecology 95, 2974–2983 (2014).
Mitchell, S. J. Wind as a natural disturbance agent in forests: A synthesis. Forestry 86, 147–157 (2013).
Kamimura, K., Kitagawa, K., Saito, S. & Mizunaga, H. Root anchorage of hinoki (Chamaecyparis obtuse (Sieb. Et Zucc.) Endl.) under the combined loading of wind and rapidly supplied water on soil: analyses based on tree-pulling experiments. Eur. J. For. Res. 131, 219–227 (2012).
Lugo, A. E. Visible and invisible effects of hurricanes on forest ecosystems: An international review. Austral Ecol. 33, 368–398 (2008).
Xi, W. Synergistic effects of tropical cyclones on forest ecosystems: a global synthesis. Journal of Forestry Research 26, 1–21 (2015).
Bellingham, P. J. et al. Hurricanes need not causes high mortality: the effects of Hurricane Gilbert on forest in Jamaica. J. Trop. Ecol. 8, 217–223 (1992).
Imbert, D., Labbe, P. & Rousteau, A. Hurrican Damage and Forest Structure in Guadeloupe, French West Indies. J. Trop. Ecol. 12, 663–680 (1996).
Chambers, J. Q. et al. The steady-state mosaic of disturbance and succession across an old-growth Central Amazon forest landscape. Proc. Natl. Acad. Sci. 110, 3949–3954 (2013).
Grimbacher, P. S., Catterall, C. P. & Stork, N. E. Do edge effects increase the susceptibility of rainforest fragments to structural damage resulting from a severe tropical cyclone? Austral Ecol. 33, 525–531 (2008).
McMahon, S. M., Arellano, G. & Davies, S. J. The importance and challenges of detecting changes in forest mortality rates. Ecosphere 10, e02615 (2019).
Drusch, M. et al. Sentinel-2: ESA’s Optical High-Resolution Mission for GMES Operational Services. Remote Sens. Environ. 120, 25–36 (2012).
Chambers, J. Q. et al. Hurricane Katrina’s carbon footprint on U.S. Gulf Coast forests. Science (80-.). 318, 1107 (2007).
Rifai, S. W. et al. Landscape-scale consequences of differential tree mortality from catastrophic wind disturbance in the Amazon. Ecol. Appl. 26, 2225–2237 (2016).
Schwartz, N. B. et al. Fragmentation increases wind disturbance impacts on forest structure and carbon stocks in a western Amazonian landscape. Ecol. Appl. 27, 1901–1915 (2017).
Negrón-Juárez, R. I. et al. Widespread Amazon forest tree mortality from a single cross-basin squall line event. Geophys. Res. Lett. 37, 1–5 (2010).
Breiman, L. E. O. Random Forests. 5–32 (2001).
Ishwaran, H. & Kogalur, U. B. randomForestSRC: Random Forests for Survival, Regression, and Classificatio. (RF-SRC), R Packag. version 2.7.0., https://doi.org/10.1001/jamapsychiatry.2013.1944 (2018).
Ehrlinger, J. ggRandomForests: Random Forests for Regression (2014).
Walker, L. R. Tree Damage and Recovery From Hurricane Hugo in Luquillo Experimental Forest, Puerto Rico. Biotropica 23, 379 (1991).
Walker, L. R. Timing of post-hurricane tree mortality in Puerto Rico. J. Trop. Ecol. 11, 315–320 (1995).
Bellingham, P. J., Tanner, E. V. J. & Healey, J. R. Sprouting of Trees in Jamaican Montane Forests, after a Hurricane. J. Ecol. 82, 747–758 (1994).
Lin, T. C. et al. Influence of typhoon disturbances on the understory light regime and stand dynamics of a subtropical rain forest in northeastern Taiwan. J. For. Res. 8, 139–145 (2003).
Lugo, A. E. & Scatena, F. N. Background and Catastrophic Tree Mortality in Tropical Moist, Wet, and Rain Forests. Biotropica 28, 585 (1996).
Uriarte, M., Thompson, J. & Zimmerman, J. K. Hurricane María tripled stem breaks and doubled tree mortality relative to other major storms. Nat. Commun. 10, 1362 (2019).
Paine, R. T., Tegner, M. J. & Johnson, E. A. Compounded Perturbations Yield Ecological Surprises. Ecosystems 1, 535–545 (1998).
Uriarte, M. et al. Natural disturbance and human land use as determinants of tropical forest dynamics: Results from a forest simulator. Ecol. Monogr. 79, 423–443 (2009).
Clark, J. S. et al. Ecological forecasts: an emerging imperative. Science 293, 657–60 (2001).
Zimmerman, J. K. et al. Responses of Tree Species to Hurricane Winds in Subtropical Wet Forest in Puerto-Rico – Implications for Tropical Tree Life- Histories. J. Ecol. 82, 911–922 (1994).
Wang, F. & Xu, Y. J. Comparison of remote sensing change detection techniques for assessing hurricane damage to forests. Environ. Monit. Assess. 162, 311–326 (2010).
Van Beusekom, A. E., Álvarez-Berríos, N. L., Gould, W. A., Quiñones, M. & González, G. Hurricane Maria in the U.S. Caribbean: Disturbance forces, variation of effects, and implications for future storms. Remote Sens. 10, 1–14 (2018).
Zhang, X., Wang, Y., Jiang, H. & Wang, X. Remote-sensing assessment of forest damage by Typhoon Saomai and its related factors at landscape scale. Int. J. Remote Sens. 34, 7874–7886 (2013).
Hu, T. & Smith, R. B. The impact of Hurricane Maria on the vegetation of Dominica and Puerto Rico using multispectral remote sensing. Remote Sens. 10, (2018).
Hoque, M. A. A., Phinn, S. & Roelfsema, C. A systematic review of tropical cyclone disaster management research using remote sensing and spatial analysis. Ocean Coast. Manag. 146, 109–120 (2017).
Batke, S. P., Jocque, M. & Kelly, D. L. Modelling hurricane exposure and wind speed on a mesoclimate scale: A case study from Cusuco NP, Honduras. PLoS One 9, (2014).
Batke, S. P. & Kelly, D. L. Tree damage and microclimate of forest canopies along a hurricane-impact gradient in Cusuco National Park, Honduras. J. Trop. Ecol. 30, 457–467 (2014).
Foster, D. & Boose, E. Patterns of Forest Damage Resulting from Catastrophic Wind in Central New England, USA. Br. Ecol. Soc. 80, 79–98 (1992).
Xi, W. & Peet, R. K. Hurricane effects on the piedmont forests: Patterns and implications. Ecol. Restor. 26, 295–298 (2008).
Luke, D., McLaren, K. & Wilson, B. Modeling Hurricane Exposure in a Caribbean Lower Montane Tropical Wet Forest: The Effects of Frequent, Intermediate Disturbances and Topography on Forest Structural Dynamics and Composition. Ecosystems 19, 1178–1195 (2016).
Xi, W. Forest response to natural disturbance: Change in structure and diversity on a North Carolina Piedmont forest in response to catastrophic wind events. 368 (2005).
Xi, W., Peet, R. K., Decoster, J. K. & Urban, D. L. Tree damage risk factors associated with large, infrequent wind disturbances of Carolina forests. Forestry 81, 317–334 (2008).
Scatena, F. N. & Lugo, A. E. Geomorphology, disturbance, and the vegetation and soils of two subtropical wet steep land watersheds in Puerto Rico. Geomorphology 13, 199–213 (1995).
Pasch, R. J., Penny, A. B. & Berg, R. Hurricane Maria. National Hurricane Center Tropical Cyclone Report (2019). AL142016
Keellings, D. & Hernández Ayala, J. J. Extreme Rainfall Associated With Hurricane Maria Over Puerto Rico and Its Connections to Climate Variability and Change. Geophys. Res. Lett. 46, 2964–2973 (2019).
Bessette-Kirton, E. K. et al. Map Data Showing Concentration of Landslides Caused by Hurricane Maria in Puerto Rico. (2017).
Larsen, M. C. & Simon, A. A rainfall intensity-duration threshold for landslides in a humid- tropical environment, Puerto Rico. Geogr. Ann. Ser. A 75 A, 13–23 (1993).
Miller, G. L. & Lugo, A. E. Guide to the ecological systems of Puerto Rico. Gen. Tech. Rep. IITF-GTR-35 1–436 (2009).
Daly, C., Helmer, E. H. & Quinones, M. Mapping the climate of Puerto Rico, Vieques and Culebra. Int. J. Climatol. 23, 1359–1381 (2003).
Kennaway, T. & Helmer, E. The Forest Types and Ages Cleared for Land Development in Puerto Rico. GIScience Remote Sens. 44, 356–382 (2007).
Helmer, E. H., Brandeis, T. J., Lugo, A. E. & Kennaway, T. Factors influencing spatial pattern in tropical forest clearance and stand age: Implications for carbon storage and species diversity. J. Geophys. Res. Biogeosciences 113, 1–14 (2008).
Hansen, M. C. et al. High-Resolution Global Maps of. Science (80-.). 342, 850–853 (2013).
Schwartz, N. B., Budsock, A. M. & Uriarte, M. Fragmentation, forest structure, and topography modulate impacts of drought in a tropical forest landscape. Ecology 100, (2019).
Muscarella, R., Uriarte, M., Erickson, D. L. & Swenson, N. G. Climate and Biodiversity Effects on Standing Biomass in Puerto Rican Forests. Caribb. Nat. 199–217 (2016).
Barone, J. A., Thomlinson, J., Cordero, P. A. & Zimmerman, J. K. Metacommunity structure of tropical forest along an elevation gradient in Puerto Rico. J. Trop. Ecol. 24, 525–534 (2008).
Thompson, J. et al. Land Use History, Environment, and Tree Composition in a Tropical Forest. Ecol. Appl. 12, 1344–1363 (2002).
TIGER/Line Shapefile, 2016, Series Information for the Current Census Tract State-based Shapefile. (2016).
CRIM, P. Hydrography Revision, 2001-04 from CRIM Basemap, scale 1:5000, Aguas Buenas, PR Quadrangle. (2006).
Gorelick, N. et al. Google Earth Engine: Planetary-scale geospatial analysis for everyone. Remote Sens. Environ. 202, 18–27 (2016).
Adams, J. B. & Gillespie, A. R. Remote sensing of landscapes with spectral images: A physical modeling approach. Remote Sensing of Landscapes with Spectral Images: A Physical Modeling Approach https://doi.org/10.1017/CBO9780511617195 (2006).
Canham, C. D., Thompson, J., Zimmerman, J. K. & Uriarte, M. Variation in susceptibility to hurricane damage as a function of storm intensity in puerto Rican tree species. Biotropica 42, 87–94 (2010).
Scatena, F. N., Silver, W., Siccama, T., Johnson, A. & Sanchez, M. J. Biomass and Nutrient Content of the Bisley Experimental Watersheds, Luquillo Experimental Forest, Puerto Rico, Before and After Hurricane Hugo, 1989. Biotropica 25, 15–27 (1993).
Carswell, W. J. The 3D Elevation Program: summary for Puerto Rico. https://doi.org/10.3133/fs20133097 (2016).
Brown, S. Estimating biomass and biomass change of tropical forests: a primer. (Food and Agriculture Organization of the United Nations, 1986).
Metcalf, E. J. C., Clark, J. S. & Clark, D. A. Tree growth inference and prediction when the point of measurement changes: modelling around buttresses in tropical forests. J. Trop. Ecol. 25, 1–12 (2009).
Cushman, K. C., Muller-Landau, H. C., Condit, R. S. & Hubbell, S. P. Improving estimates of biomass change in buttressed trees using tree taper models. Methods Ecol. Evol. 5, 573–582 (2014).
Mark D. Powell, Sam H. Houston, Luis R. Amat, Nirva Morisseau-Leroy, The HRD real-time hurricane wind analysis system. Journal of Wind Engineering and Industrial Aerodynamics 77–78, 53–64 (1998).
Emery R. Boose, David R. Foster, Marcheterre Fluet. Hurricane Impacts to Tropical and Temperate Forest Landscapes. Ecological Monographs 64(4), 369–400 (1994).
Reed, J. C. & Bush, C. A. About the geologic map in the National Atlas of the United States of America. (U.S. Geological Survey, 2007).
Hijmans, R. J. et al. Raster: raster: Geographic data analysis and modeling. R package, version (2011).
Cutler, R. et al. Random Forests for Classification in Ecology. 88, 2783–2792 (2007).
Friedman, J. H. & Popescu, B. E. Predictive learning via rule ensembles. Ann. Appl. Stat. 2, 916–954 (2008).
Fokkema, M. & Christoffersen, B. Prediction Rule Ensembles Version. 0.7.1, (2019).
Molnar, C. iml: An R package for Interpretable Machine Learning. J. Open Source Softw. 3, 786 (2018).
Source: Ecology - nature.com