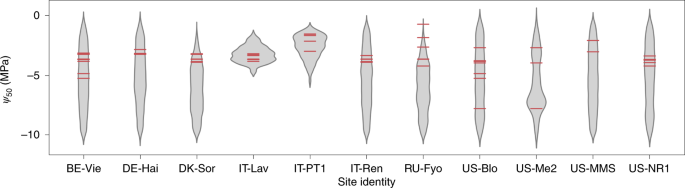
Oki, T. & Kanae, S. Global hydrological cycles and world water resources. Science 313, 1068–1072 (2006).
Seager, R. et al. Projections of declining surface-water availability for the southwestern United States. Nat. Clim. Change 3, 482–486 (2013).
Good, S. P., Noone, D. & Bowen, G. Hydrologic connectivity constrains partitioning of global terrestrial water fluxes. Science 349, 175–177 (2015).
Trugman, A., Medvigy, D., Mankin, J. & Anderegg, W. Soil moisture stress as a major driver of carbon cycle uncertainty. Geophys. Res. Lett. 45, 6495–6503 (2018).
Green, J. K. et al. Large influence of soil moisture on long-term terrestrial carbon uptake. Nature 565, 476–479 (2019).
Konings, A., Williams, A. & Gentine, P. Sensitivity of grassland productivity to aridity controlled by stomatal and xylem regulation. Nat. Geosci. 10, 284–289 (2017).
Rigden, A. J. & Salvucci, G. D. Stomatal response to humidity and CO2 implicated in recent decline in US evaporation. Global Change Biol. 23, 1140–1151 (2017).
Mirfenderesgi, G. et al. Tree level hydrodynamic approach for resolving aboveground water storage and stomatal conductance and modeling the effects of tree hydraulic strategy. J. Geophys. Res. Biogeosci. 121, 1792–1813 (2016).
Reichstein, M. et al. Climate extremes and the carbon cycle. Nature 500, 287–295 (2013).
Novick, K. A. et al. The increasing importance of atmospheric demand for ecosystem water and carbon fluxes. Nat. Clim. Change 6, 1023–1027 (2016).
IPCC Climate Change 2013: The Physical Science Basis (eds Stocker, T. F. et al.) (Cambridge Univ. Press, 2013).
Tyree, M. T. & Sperry, J. S. Vulnerability of xylem to cavitation and embolism. Ann. Rev. Plant Biol. 40, 19–36 (1989).
Anderegg, W. R. et al. Hydraulic diversity of forests regulates ecosystem resilience during drought. Nature 561, 538–541 (2018).
Feng, X., Dawson, T. E., Ackerly, D. D., Santiago, L. S. & Thompson, S. E. Reconciling seasonal hydraulic risk and plant water use through probabilistic soil–plant dynamics. Global Change Biol. 23, 3758–3769 (2017).
Oleson, K. W. et al. Technical Description of Version 4.5 of the Community Land Model (CLM) NCAR Technical Note NCAR/TN-503+STR (National Center for Atmospheric Research, 2013).
Milly, P. C. et al. An enhanced model of land water and energy for global hydrologic and earth-system studies. J. Hydrometeorol. 15, 1739–1761 (2014).
Bonan, G., Williams, M., Fisher, R. & Oleson, K. Modeling stomatal conductance in the earth system: linking leaf water-use efficiency and water transport along the soil–plant–atmosphere continuum. Geosci. Model Dev. 7, 2193–2222 (2014).
Anderegg, W. R. et al. Plant water potential improves prediction of empirical stomatal models. PloS ONE 12, e0185481 (2017).
Anderegg, W. R. Spatial and temporal variation in plant hydraulic traits and their relevance for climate change impacts on vegetation. New Phytol. 205, 1008–1014 (2015).
Meinzer, F. C., McCulloh, K. A., Lachenbruch, B., Woodruff, D. R. & Johnson, D. M. The blind men and the elephant: the impact of context and scale in evaluating conflicts between plant hydraulic safety and efficiency. Oecologia 164, 287–296 (2010).
Katul, G. G., Palmroth, S. & Oren, R. Leaf stomatal responses to vapour pressure deficit under current and CO2-enriched atmosphere explained by the economics of gas exchange. Plant Cell Environ. 32, 968–979 (2009).
Manzoni, S. et al. Optimizing stomatal conductance for maximum carbon gain under water stress: a meta-analysis across plant functional types and climates. Funct. Ecol. 25, 456–467 (2011).
Mrad, A. et al. A dynamic optimality principle for water use strategies explains isohydric to anisohydric plant responses to drought. Front. For. Global Change 2, 49 (2019).
Oren, R. et al. Survey and synthesis of intra- and interspecific variation in stomatal sensitivity to vapour pressure deficit. Plant Cell Environ. 22, 1515–1526 (1999).
Mrad, A., Domec, J.-C., Huang, C.-W., Lens, F. & Katul, G. A network model links wood anatomy to xylem tissue hydraulic behaviour and vulnerability to cavitation. Plant Cell Environ. 41, 2718–2730 (2018).
Venturas, M. D., Sperry, J. S. & Hacke, U. G. Plant xylem hydraulics: what we understand, current research, and future challenges. J. Integr. Plant Biol. 59, 356–389 (2017).
Doughty, C. E. et al. Drought impact on forest carbon dynamics and fluxes in Amazonia. Nature 519, 78–82 (2015).
Fisher, R. A. et al. Vegetation demographics in Earth system models: a review of progress and priorities. Global Change Biol. 24, 35–54 (2018).
Eller, C. B. et al. Modelling tropical forest responses to drought and El Niño with a stomatal optimization model based on xylem hydraulics. Phil. Trans. R. Soc. B 373, 20170315 (2018).
Kennedy, D. et al. Implementing plant hydraulics in the community land model, version 5. J. Adv. Model. Earth Syst. 11, 485–513 (2019).
Liu, Y. et al. Increasing atmospheric humidity and CO2 concentration alleviate forest mortality risk. Proc. Natl Acad. Sci. USA 114, 9918–9923 (2017).
Katul, G., Manzoni, S., Palmroth, S. & Oren, R. A stomatal optimization theory to describe the effects of atmospheric CO2 on leaf photosynthesis and transpiration. Ann. Bot. 105, 431–442 (2009).
Farquhar, G. D., Caemmerer, S. V. & Berry, J. A. A biochemical model of photosynthetic CO2 assimilation in leaves of C3 species. Planta 149, 78–90 (1980).
Huang, C.-W. et al. The effect of plant water storage on water fluxes within the coupled soil–plant system. New Phytol. 213, 1093–1106 (2017).
Cowan, I. & Farquhar, G. Stomatal function in relation to leaf metabolism and environment. Symp. Soc. Exp. Biol. 31, 471–505 (1977).
Hari, P., Mäkelä, A., Korpilahti, E. & Holmberg, M. Optimal control of gas exchange. Tree Physiol. 2, 169–175 (1986).
Medlyn, B. E. et al. Reconciling the optimal and empirical approaches to modelling stomatal conductance. Global Change Biol. 17, 2134–2144 (2011).
Sperry, J. S. et al. Predicting stomatal responses to the environment from the optimization of photosynthetic gain and hydraulic cost. Plant Cell Environ. 40, 816–830 (2017).
Manzoni, S., Vico, G., Porporato, A. & Katul, G. Biological constraints on water transport in the soil–plant–atmosphere system. Adv. Water Resourc. 51, 292–304 (2013).
Clapp, R. B. & Hornberger, G. M. Empirical equations for some soil hydraulic properties. Water Resourc. Res. 14, 601–604 (1978).
Katul, G., Leuning, R. & Oren, R. Relationship between plant hydraulic and biochemical properties derived from a steady–state coupled water and carbon transport model. Plant Cell Environ. 26, 339–350 (2003).
FLUXNET 2015 Tier 1 Dataset (FLUXNET, accessed 25 July 2018); http://fluxnet.fluxdata.org/data/fluxnet2015-dataset
Myneni, R., Knyazikhin, Y. & Park, T. MCD15A3H MODIS/Terra+Aqua Leaf Area Index/FPAR 4-day L4 Global 500 m SIN Grid V006 (NASA EOSDIS Land Processes DAAC, accessed 21 January 2019); https://doi.org/10.5067/MODIS/MCD15A3H.006
Ukkola, A. M., Haughton, N., Kauwe, M. G. D., Abramowitz, G. & Pitman, A. J. FluxnetLSM R package (v1. 0): a community tool for processing FLUXNET data for use in land surface modelling. Geosci. Model Develop. 10, 3379–3390 (2017).
Healey, S. et al. CMS: GLAS LiDAR-derived Global Estimates of Forest Canopy Height, 2004–2008 (ORNL DAAC, accessed 21 January 2019); https://doi.org/10.3334/ORNLDAAC/1271
Fan, Y., Miguez-Macho, G., Jobbágy, E. G., Jackson, R. B. & Otero-Casal, C. Hydrologic regulation of plant rooting depth. Proc. Natl Acad. Sci. USA 114, 10572–10577 (2017).
Jackson, R. et al. A global analysis of root distributions for terrestrial biomes. Oecologia 108, 389–411 (1996).
Kottek, M., Grieser, J., Beck, C., Rudolf, B. & Rubel, F. World map of the Köppen–Geiger climate classification updated. Meteorol. Z. 15, 259–263 (2006).
Harmonized World Soil Database Version 1.2 (FAO, accessed 22 June 2016); http://www.fao.org/soils-portal
Thompson, S. E. et al. Comparative hydrology across AmeriFlux sites: the variable roles of climate, vegetation, and groundwater. Water Resourc. Res. 47, W00J07 (2011).
Kattge, J. et al. TRY—a global database of plant traits. Global Change Biol. 17, 2905–2935 (2011).
Martin-StPaul, N., Delzon, S. & Cochard, H. Plant resistance to drought depends on timely stomatal closure. Ecol. Lett. 20, 1437–1447 (2017).
Ji, C. & Schmidler, S. C. Adaptive Markov Chain Monte Carlo for Bayesian variable selection. J. Comput. Graph. Stat. 22, 708–728 (2013).
Brooks, S. P. & Gelman, A. General methods for monitoring convergence of iterative simulations. J. Graph. Stat. 7, 434–455 (1998).
Source: Ecology - nature.com